RetinaGAN
Synthesising Retina Fundus Images with GANs
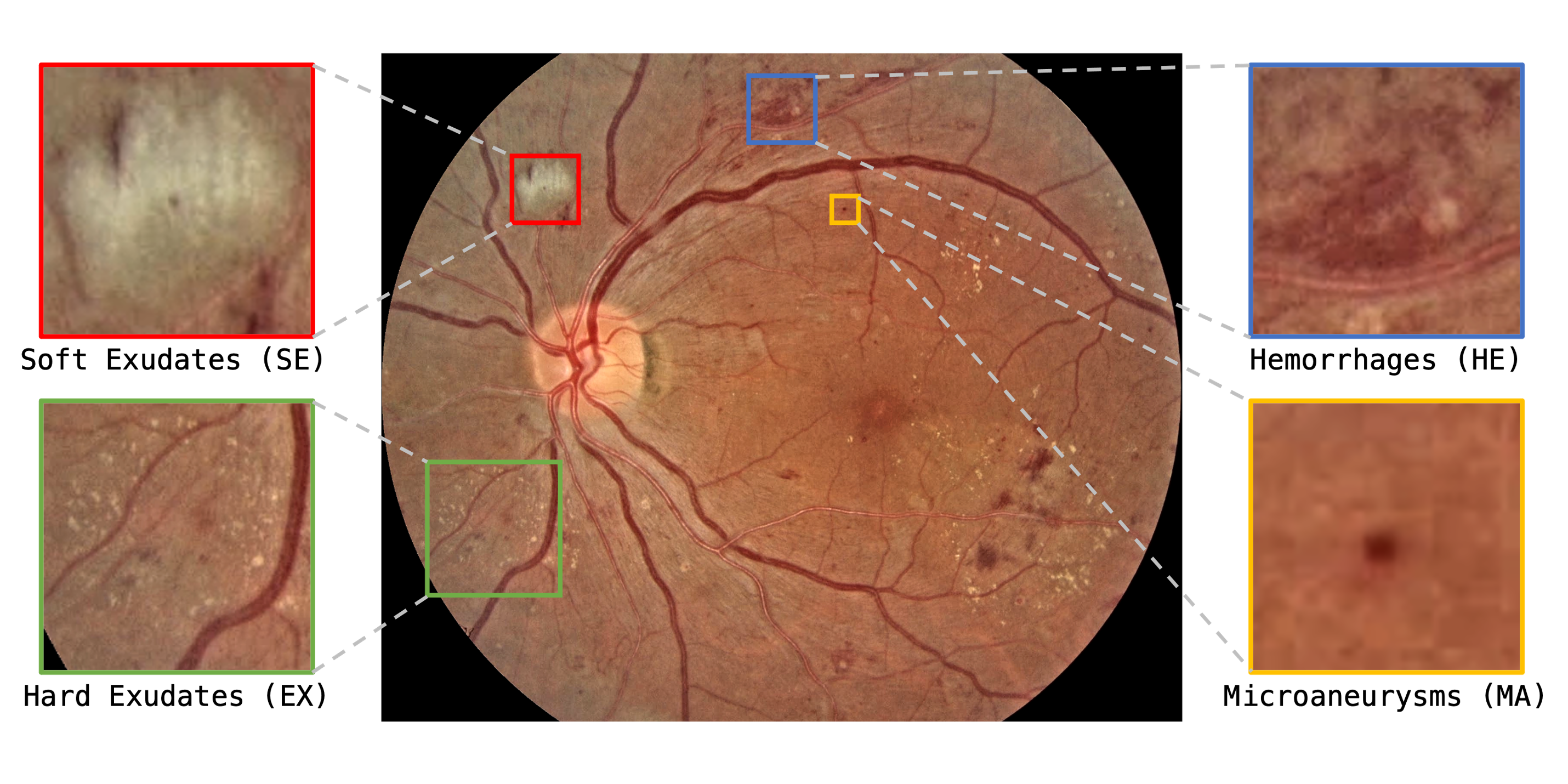
Project Details
- Publication : Biomedical Optics Express
- Date Published : 4th January 2023
- DOI : 10.1364/BOE.477906
- Code : Github
- Demo : Streamlit
High-Fidelity Diabetic Retina Fundus Image Synthesis from Freestyle Lesion Maps
Retina fundus imaging for diagnosing Diabetic Retinopathy (DR) is an efficient and patient-friendly modality, where many high-resolution images can be easily obtained for accurate diagnosis. With the advancements of deep learning, data-driven models may facilitate the process of high-throughput diagnosis especially in areas with less availability of certified human experts. Many datasets of DR already exist for training learning-based models. However, most are often unbalanced, do not have a large enough sample count, or both. This paper proposes a two-stage pipeline for generating photo-realistic retinal fundus images based on either artificially generated or free-hand drawn semantic lesion maps. The first stage uses a Conditional StyleGAN to generate synthetic lesion maps based on a DR severity grade. The second stage then uses GauGAN to convert the synthetic lesion maps into high resolution fundus images. We evaluate the photo-realism of generated images using the Fréchet Inception Distance (FID), and show the efficacy of our pipeline through downstream tasks, such as; dataset augmentation for automatic DR grading and lesion segmentation.